Knowledge is a special kind of belief, and the science of statistics provides one approach to gaining knowledge. So does faith have any direct connection to statistics? [1]
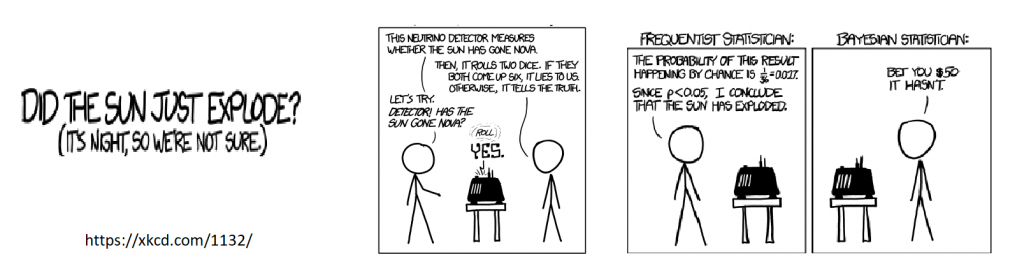
A couple of months ago Andi Wang wrote about his interest in statistics as a Christian, and now I want to share something of mine. I’m an applied statistician, using statistical methods to draw inferences from ecological, and more recently financial, data. My fascination with stats started on an ecology field trip that was part of my A-level Biology course, when an introduction to some basic statistical tests one evening revealed how we can discern scientific order in the apparent chaos of our natural environment. My fascination has gradually developed ever since – but recently I’ve been excited about ways in which faith might guide statistical practices. Drawing conclusions from numerical data, I now see, is not the purely objective process it might seem.
The basic point to make is that data don’t speak. Although we talk of “analysing” data as if conclusions were latent in the data, waiting to be released, that’s far from an accurate portrayal. We also proclaim what data “tell” us and speak of “following the evidence where it leads” – all of which, it can be argued, are seriously misleading. In fact we inevitably (1) collect data according to prior assumptions, (2) bring our beliefs to bear when choosing statistical methods and (3) incorporate theoretical ideas into our analyses.
That’s the simple point I want to make against the objectivity of statistical inference. To argue for a specifically religious factor, I need to give a simple example and recommend a book that makes the case more fully.
Let’s take a very simple problem: what’s the probability that this coin will land heads up? Here’s a good empirical method: toss the coin 100 times and record the number of “heads”. We can easily imagine doing this and getting, say, 47 heads and 53 tails. So we might conclude that the coin has a 47% chance of landing heads. Statisticians call this the maximum-likelihood estimate of the coin’s probability of landing heads up: this value would make our observations more likely than would any other value – including 50%. (Philosophers call this general approach “inference to the best explanation”.)
Fortunately there’s another method: do a null-hypothesis test based on a 50% “null hypothesis” (a default, “nothing-going-on” starting point). Then having got 47 heads, we’d refer to a table of probabilities for possible outcomes from 100 trials with 50% probability in each one to find the chance of getting a number of heads that’s at least as far away from 50 as is 47 (this needs a bit more explanation, but it’s not the most important point). The answer (called our P-value) would be about 0.55 and we’d say that nothing terribly unlikely has happened if the fair-coin null hypothesis were true… whereas if we ended up with a P-value of just 0.05, we should suspect the coin of not being perfectly fair (which would mean getting fewer than 40 heads or tails). But we have two important queries: where did our null hypothesis come from, and how does that “if…whereas” line of reasoning about P-values work? The null hypothesis was based on a simple theory about coins, which means our statistical method is not as purely objective as we might have thought: for more interesting questions, the key theory might be controversial and open to change. And the P-value reasoning turns out to be based on some odd logic. For now let’s note how non-intuitive it is, and that it seems odd to derive probabilities directly from data. For more detail, see the book recommendation below!
So there we have two methods for investigating the flipping-properties of a coin, each with some obvious problems. Regarding my three concerns above, we may have (1) chosen a flipping technique which we believe to be unbiased (but further research[2] could call it into question); (2) chosen the null-hypothesis method, unperturbed by caricatures like the cartoon above; and (3) employed the simple theory that coins are equally likely to land heads up as tails. But what about the religious factor?
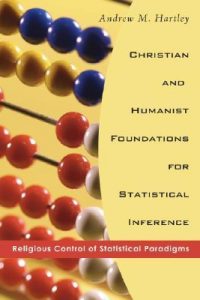
The religious issue, actually, is right there. Humanist–empiricist views of science require that knowledge can arise objectively from evidence without dependence on prior beliefs, so that truths can be established independently of each other, free of subjection to historical, social and cultural factors – especially religious ones! – ensuring the resulting ‘knowledge’ can be divorced from other beliefs. A Christian view[3], by contrast, might insist that knowledge is a form of belief, and that beliefs can never be reduced to data. The particulars of data – including all kinds of experience – shape our beliefs, and that’s how we grow our knowledge. What’s more, there is an alternative statistical approach to those outlined above that explicitly builds conclusions upon prior beliefs: Bayesian inference. For a much better exposition, I can heartily recommend the wonderfully-titled “Christian and Humanist Foundations for Statistical Inference” by Andrew Hartley.
_____________________________________________
[1] For enlightened nerdy insight about the above cartoon, see this discussion.
[2] e.g. Diaconis et al. (2007) SIAM Review 49:211-235.
[3] I refer especially to the Reformed and Reformational traditions.
- Artificial thinking? - April 2, 2025
- A degree of critical thinking - September 2, 2024
- Reforming Economics? - July 3, 2024